Understanding the Difference Between Structured and Unstructured Data
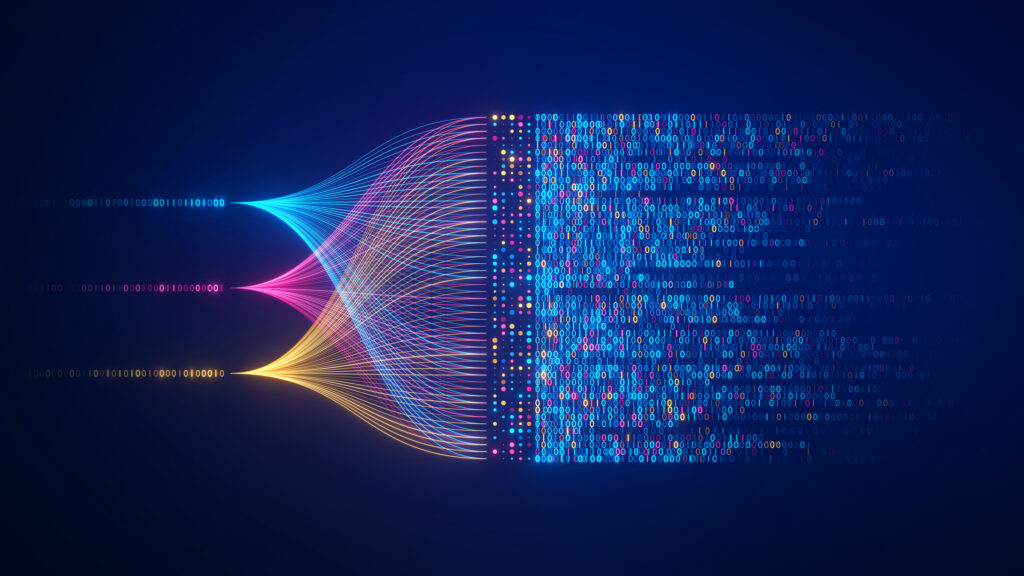
In the age of data-informed decision-making, distinguishing between structured and unstructured data is essential for optimal data management and processing strategies. Structured and unstructured data underpin various analytical workflows, from predictive models to customer insights. While structured data is organized into predefined schemas of rows and columns, unstructured data—encompassing formats such as text, images, and video—lacks such a structure, posing distinct challenges and opportunities.
For example, healthcare organizations use structured data, like patient records, alongside unstructured data from medical imaging or patient feedback to improve patient outcomes. Similarly, retailers analyze unstructured customer reviews to complement structured sales data, identifying trends and preferences that inform marketing and inventory decisions. As unstructured data now comprises over 80% to 90% of all generated information, businesses must implement advanced techniques to harness its potential while maintaining efficient structured data management.
What is Structured Data?
Structured data refers to highly organized, machine-readable data that follows a strict schema or data model, making it easily searchable and analyzable. It is essential for business intelligence, operational reporting, and ensuring data readiness for AI workflows. Common formats include relational databases (e.g., SQL), CSV files, XML, and JSON, where data is systematically stored in rows and columns. This rigid structure enables efficient storage, retrieval, and analysis, playing a pivotal role in machine learning (ML), artificial intelligence (AI), and data visualization systems.
Structured data ensures high AI data quality by providing labeled and organized datasets, which is necessary for training accurate predictive models. For example, AI systems can rapidly process structured datasets such as sales figures, inventory metrics, or transaction logs to inform business decisions, leveraging structured data for accurate and scalable models.
What is Unstructured Data?
Unstructured data lacks a predefined schema, making searching, processing, and analyzing more difficult. This data type includes diverse forms such as text, images, audio, and social media content, often stored in its raw format. Advanced techniques like Natural Language Processing (NLP), machine learning, and data mining are required to process and derive insights from unstructured data.
Because of its complexity, unstructured data poses challenges for AI data quality, as AI models need significant preprocessing, such as tagging and organizing, to ensure data readiness for AI. For instance, raw customer feedback on social media requires sentiment analysis and keyword extraction before AI can understand it.
Despite these challenges, unstructured data is invaluable for gaining qualitative insights and uncovering deeper trends that structured data alone cannot reveal. With the proper techniques, businesses can harness unstructured data to drive innovation and improve customer experience.
What is the Difference Between Structured and Unstructured Data?
Three crucial factors emerge when examining the key differences between structured and unstructured data: data organization and format, ease of analysis and processing, and scalability and storage requirements.
1. Data Organization and Format
Structured data is organized in a predefined schema, typically stored in tables with rows and columns, allowing for predictable, consistent data storage. Relational databases like SQL use this structure for easy data retrieval and querying.
Unstructured data, on the other hand, lacks this uniform organization. It can exist in a wide range of formats, from text to multimedia, which makes storage and retrieval more complex. Due to their flexibility, NoSQL databases and data lakes are typically used to store unstructured data.
2. Ease of Analysis and Processing
Processing structured data using traditional tools like SQL and rule-based analytics is relatively straightforward. Its rigid format allows for efficient searching and querying, making it ideal for routine business tasks.
Unstructured data requires advanced techniques such as NLP, machine learning, and deep learning algorithms to extract insights. Processing unstructured data, like text documents or multimedia files, can involve complex tasks like image recognition, sentiment analysis, or keyword extraction, requiring more computational power and sophisticated tools.
3. Scalability and Storage Requirements
Structured data is compact and efficient in storage, especially when schema constraints are consistent. However, its scalability can become limited as data volume grows.
Unstructured data demands larger storage systems, such as NoSQL databases or data lakes, to handle its variety and volume. These systems provide the necessary flexibility but introduce more complexity in managing data at scale.
Leveraging Structured and Unstructured Data in Knowledge Management
Both structured and unstructured data play critical roles in Knowledge Management (KM). Structured data is vital to operational efficiency, allowing businesses to automate reporting, track performance, and process clean, organized data. On the other hand, unstructured data is essential for capturing qualitative insights—tacit knowledge, customer sentiment, and collaboration efforts.
Structured Data in Knowledge Management
Structured data is essential for capturing well-defined quantitative information within KM systems. Data stored in CRM platforms, ERP systems, and Content Management Systems (CMS) with applied metadata can be efficiently queried and analyzed. This structured approach makes such data highly reliable for routine business processes, automated reporting, and advanced analytics.
Key Applications Utilizing Structured Data in Knowledge Management
- Data Access and Decision-Making: Structured data (e.g., customer interactions, inventory, financial transactions) is easily accessed from databases to inform business decisions and refine processes.
- Trend Analysis and Reporting: Businesses use structured data for trend analysis and performance reporting.
- Automation and Predictive Analytics: Structured data powers AI models to automate tasks such as generating reports, managing workflows, and predicting outcomes.
- Performance Tracking: Sales figures and inventory data are automatically processed and displayed in dashboards, helping decision-makers track business performance.
Unstructured Data in Knowledge Management
Unstructured data—from email correspondence to video interviews—captures the tacit knowledge and human experiences essential for innovation and decision-making. In KM, advanced tools like text mining, NLP, and machine learning help analyze this data, providing valuable insights into customer sentiment, market trends, and internal collaboration.
Key Applications Using Unstructured Data in Knowledge Management
- Tacit Knowledge Capture: Unstructured data (e.g., email correspondence, meeting notes, video interviews) stores valuable human insights and experiences, enriching AI models with nuanced, real-world data.
- Document and Multimedia Management: KM systems organize vast amounts of documents, research papers, and multimedia content, providing accessibility through advanced search features.
- Insight Mining with NLP and AI: Tools like NLP and machine learning analyze unstructured data (e.g., customer feedback) to extract sentiment and market trends for improved decision-making.
- Collaboration and Knowledge Sharing: Unstructured data (e.g., project reports and chat logs) enhances collaboration across teams through platforms like wikis, forums, and internal communication tools.
Integrating Structured and Unstructured Data in Knowledge Management
Modern Knowledge Management (KM) systems often combine structured and unstructured data to deliver more comprehensive insights. For example, a single search query could retrieve structured data (e.g., customer satisfaction scores) alongside unstructured data (e.g., customer reviews or emails), enriching the decision-making process.
AI models also benefit from this integration. Structured data, like sales figures, trains predictive models, while unstructured data, such as support tickets, can reveal recurring issues. These data types provide a fuller understanding of customer behavior, helping businesses improve products, services, and customer satisfaction.
Leveraging Structured and Unstructured Data for Comprehensive Insights
Understanding the difference between structured and unstructured data is critical for maximizing their potential in data analytics, AI, and Knowledge Management. Dr. Anthony Rhem, a leading authority in knowledge management, advises that businesses utilize both data types to support AI model accuracy and decision-making. He stresses that integrating structured and unstructured data enables companies to capture comprehensive insights and improve operational efficiency.
To remain competitive in today’s data-driven world, organizations must combine structured and unstructured data, using advanced AI tools and techniques to unlock their data’s full potential. Proper data readiness for AI is essential to successfully implementing these technologies, enabling businesses to make more informed decisions and fostering continuous innovation.
How to Organize a Knowledge Base: A Step-by-Step Guide
11 Benefits of Knowledge Management (+ KMS Advantages You Shouldn’t Miss)
Start working smarter with Bloomfire
See how Bloomfire helps companies find information, create insights, and maximize value of their most important knowledge.
Take a self guided Tour
See Bloomfire in action across several potential configurations. Imagine the potential of your team when they stop searching and start finding critical knowledge.