The Importance of Data Quality for AI
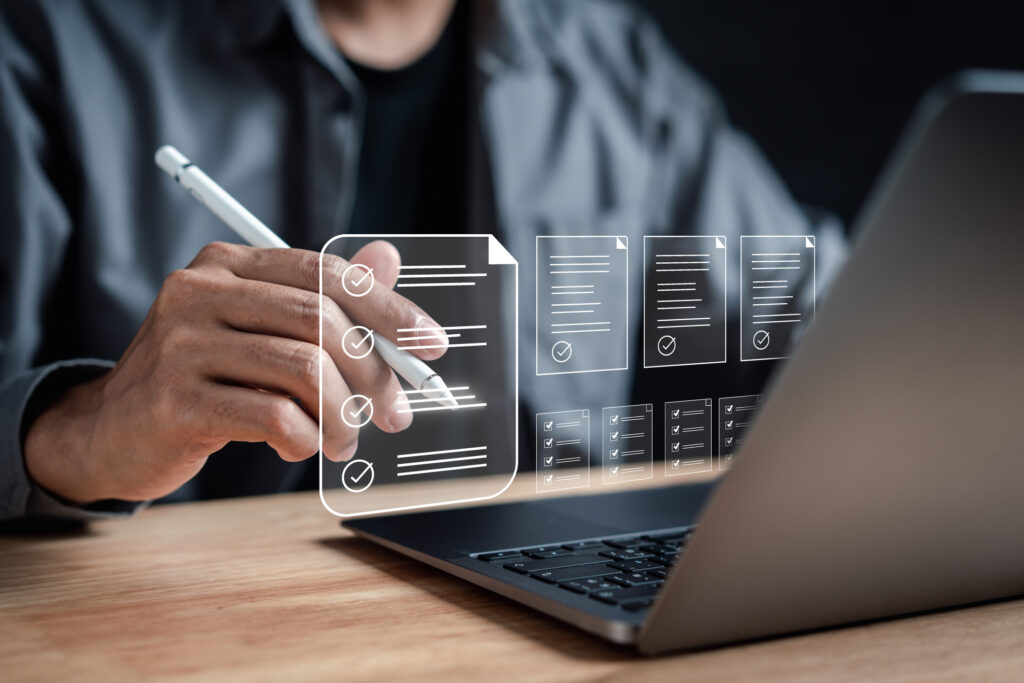
AI Data quality is paramount for ensuring accurate, unbiased, and reliable outcomes in today’s artificial intelligence-driven world. With the proliferation of diverse content such as text, images, audio, and video, the quality of this data directly influences the performance of AI and large language models (LLMs) like GPT. Although structured data (organized in clear formats like databases) has been the traditional focus, the sheer volume and diversity of unstructured knowledge now make up approximately 80-90% of all data generated globally.
Given its vast presence, unstructured content is critical in powering AI systems and LLMs. High-quality data and knowledge are essential in natural language processing (NLP) and AI systems that drive better predictions, decision-making, and insights. On the other hand, poor-quality data leads to inaccurate models, biased outputs, and missed opportunities.
This article will explore the importance of knowledge quality, focusing on the challenges, key risk factors, and best practices for improving Gen AI data quality.
The Role of Data in AI Models
Data, especially unstructured data, forms the backbone of many AI applications, particularly large language models (LLMs) like GPT and similar technologies. These models rely heavily on vast amounts of content—whether it’s text for NLP, images for computer vision, or audio and video for multimodal applications. AI systems use this content to interpret language, identify patterns, and predict outcomes.
Given the volume and diversity of unstructured knowledge, AI is critical to extracting insights from it. This allows organizations to make more informed decisions, create personalized experiences, and automate complex processes.
For example, NLP applications—such as chatbots, virtual assistants, and automated customer service platforms—depend on the quality of text data. These systems require well-prepared content to provide accurate responses, interpret sentiment, and understand user intent. Similarly, LLMs like GPT-4 use vast content sources to generate knowledge-based insights, summarize data, or answer user queries.
Ensuring the quality of this knowledge is pivotal to the success of AI systems and their ability to generate reliable and accurate outputs. The goal is to ensure that AI-driven applications, such as LLMs and NLP tools, produce trustworthy, unbiased insights that drive better decision-making, improve customer experiences, and automate processes effectively. High-quality content lays the foundation for AI accuracy. At the same time, low-quality data can lead to biased, skewed, or irrelevant outputs from AI-based applications, ultimately undermining their value to organizations.
Common Challenges in Gen AI Data Quality
Several critical risk factors, listed below, emerge when handling diverse content in AI and LLMs because poor data quality can lead to biased outputs, irrelevant conclusions, or erroneous predictions. If addressed properly, these can ensure AI models’ accuracy, reliability, and scalability.
1. Data Quality and Noise
Data often contains noise—irrelevant or redundant information. For example, text data may include slang or errors, while image data may have poor lighting or irrelevant backgrounds. AI models using noisy data are less likely to perform well, reducing their accuracy and generalization abilities.
2. Ambiguity and Contextual Dependency
The ambiguity inherent in content presents another challenge. For instance, a word like “bank” could refer to a financial institution or a riverbank. LLMs may struggle to disambiguate terms without sufficient context, leading to inaccurate predictions or irrelevant outputs.
3. Bias in the Data
Bias can creep into AI systems through content. The model may produce biased results if the dataset is skewed toward specific demographics or perspectives. For example, LLMs trained on biased content might generate biased job recommendations or discriminatory content, affecting fairness and reliability.
4. Incomplete or Fragmented Data
Content often comes in fragments. Message threads, for example, may lack context and contain only some of the information needed for a complete analysis, leading to misleading or incomplete AI predictions.
5. Data Privacy and Security Risks
Handling data such as email communications or personally identifiable information (PII) often involves private information. Safeguarding this content while ensuring AI models are trained effectively requires robust security measures.
Key Factors of Data Quality for AI
Improving knowledge base quality is critical for effectively employing AI and LLMs in knowledge management platforms, chatbots, and other AI-driven applications, ensuring optimal productivity and accurate results. In these systems, data quality directly impacts the accuracy and reliability of outputs. Ensuring that the data fed into these models is free of R.O.T – redundant, outdated, and trivial information – is essential to achieving meaningful results and operational efficiency outcomes.
Data quality is vital when leveraging knowledge management, content generation, or decision-making processes. A thorough content evaluation is an effective strategy to ensure your data is accurate, relevant, and complete. Organizations should assess the following factors of data quality for AI to ensure they are getting the best results from AI tools:
1. Knowledge Gaps and Data Completeness
Regular evaluations help uncover gaps in information that can negatively affect AI model performance. Understanding if important data is missing from your knowledge base in real-time so you can fill those gaps enhances model accuracy and prevents incomplete or erroneous predictions. This is especially important in fast-changing fields like technology, medicine, or law, where knowledge quickly changes and evolves.
2. Data Relevance and Timeliness
Keeping data relevant and timely is crucial for AI models. Without periodic reviews, models might rely on obsolete or irrelevant information, leading to inaccurate outputs. Implementing strategies to update and align data with current trends or developments regularly ensures that LLMs provide meaningful and up-to-date insights.
3. Data Accuracy and Bias
Identifying and rectifying biases or inaccuracies is essential to reduce the risk of biased outputs from skewed or incomplete data. Techniques such as data enrichment can help mitigate these risks by adding diverse and balanced information to the datasets, thereby improving AI-driven solutions’ fairness and reliability.
How RAG Pipelines Boost AI Accuracy
In addition to having high-quality knowledge and content, implementing Retrieval-Augmented Generation (RAG) pipelines enhances the ability of LLMs to provide high-quality outputs by enabling real-time retrieval of relevant information from specific knowledge sets. This method ensures that AI systems are using up-to-date, accurate, and contextually relevant information to provide answers and results to users.
RAG also helps combat issues like data drift and model hallucinations, common challenges in which models generate incorrect or outdated responses based solely on pre-trained data. Organizations can achieve relevant AI outputs and improve AI reliability by maintaining high-quality content and integrating RAG pipelines in a knowledge management platform.
Optimizing AI Data Quality for Better Results
Ensuring high-quality data is essential for the success of AI models and GenAI solutions. Organizations must adopt comprehensive strategies to improve content quality by assessing and addressing R.O.T. Techniques like Retrieval-Augmented Generation (RAG) further enhance AI’s ability to provide accurate, contextually relevant, and timely insights.
As AI evolves, focusing on data quality, information architecture, and robust knowledge audits will remain critical for driving successful and trustworthy AI solutions. By implementing these best practices, organizations can harness the full potential of AI and LLMs while mitigating risks associated with unstructured content quality.
Optimize Your Data for AI
Prepare your data & knowledge for LLMs and RAG pipelines with Bloomfire.
Learn MoreThe Knowledge Management Process: A Roadmap to a Successful Implementation Program
Knowledge Management: Why It’s Important to the Decision-Making Process
The Value of Company-Wide Knowledge Management in 2025
Start working smarter with Bloomfire
See how Bloomfire helps companies find information, create insights, and maximize value of their most important knowledge.
Take a self guided Tour
See Bloomfire in action across several potential configurations. Imagine the potential of your team when they stop searching and start finding critical knowledge.